Rapid damage assessment for earthquakes from synthetic aperture radar and machine learning
Co-supervisors: Andy Hooper (Leeds), Stuart King (Edinburgh), Sarah Douglas (SatSense Ltd)
Earthquakes strike suddenly and without warning and can impact a large geographic region. Rapid identification of the amount and distribution of damage is critical for local and international responders (1, 2). This PhD project will develop tools for rapid damage mapping following large earthquakes, exploiting recent advances in synthetic aperture radar imaging and machine learning.
Satellite observations can also be used to directly assess damage resulting from earthquakes and secondary hazards such as landslides (3). Methods that use high-resolution optical images are powerful but fail when there is cloud cover, and currently require significant human involvement to produce results; results from optical methods are typically not available on the timescales required for emergency response (4). Satellite radar (SAR) imagery can see through clouds and can be acquired night and day. The recent proliferation of SAR missions (3) means we will soon be able to obtain new SAR imagery for most earthquakes in less than 24 hours (5).
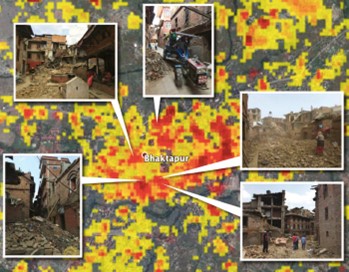
One approach that has been used to create damage-proxy maps automatically is to track changes in interferometric coherence in SAR interferograms (5, 6). These methods track changes in the scattering properties; they are powerful but have relatively low spatial resolution as the coherence is calculated from patches of pixels (Fig. 1). Spaans and Hooper (7) proposed a method (RapidSAR) that produces coherence images at the highest resolution of the satellite using stacks of pre-processed radar imagery. RapidSAR coherence maps are significantly sharper than coherence maps from conventional imagery, showing key infrastructure more clearly. However, calculating coherence requires pre-processing large stacks of radar imagery.
In COMET, we have developed a system for processing large quantities of Sentinel-1 satellite radar imagery over tectonic and volcanic areas (8). The student will exploit this to produce RapidSAR coherence, phase and amplitude time series from Sentinel-1 SAR data. They will test the use of various machine learning and deep learning classifiers (e.g., boosted decision trees, convolutional neural networks) to identify areas where rapid changes have occurred during an earthquake. This may involve use of individual observations or series of observations to construct a suitable feature set for change detection. The first step, which will involve the most research and experimentation, will be to test and develop algorithms using data from an earthquake with known distribution of damage, for example recent earthquakes in Nepal, New Zealand, and Italy. The student will then apply the method systematically to a suite of earthquakes that occur during the timeframe of the project, with the ultimate aim of implementing a routine rapid damage assessment system.
The project would suit a numerate scientist from a wide range of disciplines, including physical sciences, mathematics, and computer science. The student will receive a wide array of training in Earth Observation and Machine Learning as part of the CDT and will benefit from membership of the COMET network (http://comet.nerc.ac.uk).
References
- M. Wendelbo et al., The crisis response to the Nepal earthquake: Lessons learned. European Institute for Asian Studies. Brussels, Belgium: Author, (2016).
- D. Sanderson, B. Ramalingam, NEPAL EARTHQUAKE RESPONSE: Lessons for operational agencies. The active learning network for accountability and performance in Humanitarian action ALNAP, (2015).
- J. Elliott, R. Walters, T. Wright, The role of space-based observation in understanding and responding to active tectonics and earthquakes. Nature communications 7, 13844 (2016).
- J. G. Williams et al., Satellite-based emergency mapping using optical imagery: experience and reflections from the 2015 Nepal earthquakes. Natural hazards and earth system sciences. 18, 185-205 (2018).
- S.-H. Yun, E. J. Fielding, F. H. Webb, M. Simons. (Google Patents, 2015).
- E. J. Fielding et al., Surface ruptures and building damage of the 2003 Bam, Iran, earthquake mapped by satellite synthetic aperture radar interferometric correlation. Journal of Geophysical Research: Solid Earth 110, (2005).
- K. Spaans, A. Hooper, InSAR processing for volcano monitoring and other near‐real time applications. Journal of Geophysical Research: Solid Earth 121, 2947-2960 (2016).
- M. Lazecký et al., LiCSAR: An automatic InSAR tool for measuring and monitoring tectonic and volcanic activity. Remote Sensing 12, 2430 (2020).